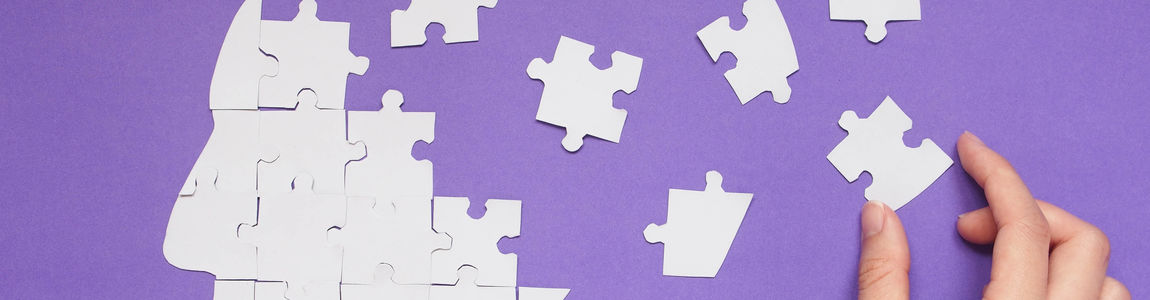
Alzheimer's: a machine learning approach to identify genetic predisposition
Alzheimer's disease is a genetic disorder caused by mutations at the level of a single gene, i.e. where a Single-Nucleotide Variant (SNV) is sufficient to cause the disorder. To date, the gene and mutation involved in the onset are known in only half of the diseases of this kind.
Several large-scale studies (called GWAS, genome-wide association studies) have uncovered information on single variants associated with a patient's propensity to develop Alzheimer's. However, SNVs cannot be used effectively for predictive purposes without considering the relationships between them and potential correlations with other genome elements.
Suffice to say, many people with a certain gene variant associated with Alzheimer's (such as a mutation in the APOE gene) do not develop the disease.
To understand how this might happen, a team of researchers from the Charles Darwin Department of Biology and Biotechnology of Sapienza University of Rome, together with the Italian Institute of Technology (IIT) and other international research centres, used a machine learning approach to analyse the entire genomic profile of patients with Alzheimer's disease and identify the genetic predispositions underlying the onset of the condition. The results of the study, conducted by the young researcher Magdalena Arnal in Gian Gaetano Tartaglia's Sapienza laboratory, have been published in the journal Alzheimer's & Dementia: Diagnosis, Assessment & Disease Monitoring.
In particular, to build the genomic profiles, a subset of data from two of the largest existing databases was used: UK Biobank and ADNI.
"There are countless possible combinations, so we developed a machine learning approach to simplify the analysis," says Magdalena Arnal. "Without this computational breakthrough, our analysis would not have been possible."
In this way, the researchers identified six SVN variants in one area of the genome, chromosome 19, which allow many individuals to be identified as potential Alzheimer's cases.
"What we discovered is called epistasis, a form of gene interaction whereby one gene variation can mask or contribute to the phenotypic expression of other genes," adds Gian Gaetano Tartaglia. "This is the key to understanding the organisation of the genome: depending on how SNVs combine, an individual may or may not develop Alzheimer's disease."
The next step for researchers will be to identify new patterns involved in other diseases.
References:
Machine learning methods applied to genotyping data capture interactions between single nucleotide variants in late onset Alzheimer's disease - Alzheimer's & Dementia: Diagnosis, Assessment & Disease Monitoring http://doi.org/10.1002/dad2.12300
Further Information
Gian Gaetano Tartaglia
Department of Biology and Biotechnology "Charles Darwin"
giangaetano.tartaglia@uniroma1.it