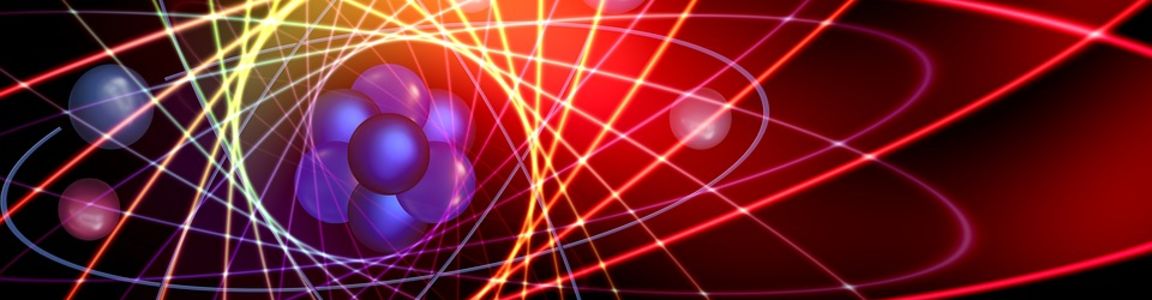
Artificial Intelligence: New Resource for the Investigation and Development of Quantum Information
In the last decade there has been a remarkable development of quantum technologies, also due to the flurry of interest of the industry towards the sectors of computing, communication and computer security.
A new study coordinated by Fabio Sciarrino, head of the Quantum Lab of the Physics Department of Sapienza University, together with the University College London, and with the contribution of the University of Texas at Austin, showed that it is possible to efficiently describe a quantum using techniques from computational learning theory, one of the most promising branches of artificial intelligence.
The results, published in the journal Science Advances, were also presented during the Quantum Week (3-6 April 2019 at Sapienza) coordinated by the Sapienza Quantum Lab, to which over 400 representatives of the quantum scientific community from all over the world took part.
In general, the description of a quantum state requires a number of parameters that grows exponentially with the number of particles involved. This poses severe limits to the possibility of describing and simulating quantum system: for example, the complete description of a quantum state composed of 40 particles would already exceed the world storage capacity.
It is, however, possible to circumvent the complexity of the description of a quantum state with new techniques: in a probabilistic sense, a number of measures that grows linearly with the number of particles is sufficient to describe it in an approximate way. This strategy, until now only theoretical, was proposed by Scott Aaronson Professor of theoretical computer science at the University of Texas at Austin. The new work, which saw the collaboration of Aaronson himself, experimentally verified this principle for the first time on a photonic platform.
"Our results," states Andrea Rocchetto of University College London, first author of the article, "demonstrate the effectiveness of the computational learning theory applied to quantum information by providing experimental evidence that quantum states can be roughly described with a number of measures that scales linearly with the number of qubits (the quantum information unit)."
"This result," explains Fabio Sciarrino, "has profound implications on our ability to model quantum systems: machine learning represents a promising resource for the development of experimental quantum information and is a valuable and widely used tool to reduce the complexity of physical models. However, often," continues Sciarrino, "It is not clear to what extent the results of a learning algorithm can be trusted. The techniques that we have implemented instead, in addition to greatly facilitating the analysis of the data, guarantee the accuracy of the description of the system."
The next step is to exploit this methodology in the study of many-body and entangled states of complex systems in chemistry, matter physics and quantum information. "Our work," concludes Davide Poderini, PhD student of the Department of Physics, "not only offers a different point of view on over the problem of the complexity of quantum states, but in perspective also represents a tool of great value for the experimental analysis of large quantum systems."
References:
Experimental learning of quantum states - Rocchetto A., Aaronson, S., Severini, S., Carvacho, G., Poderini, D., Agresti, I., Bentivegna, M., Sciarrino, F. - Science Advances 2019, 5. DOI: 10.1126/sciadv.aau1946
Further Information
Fabio Sciarrino
Department of Physics, Sapienza University of Rome
fabio.sciarrino@uniroma1.it